Blogs / Trendy Tech Talks / What is Multimodal Artificial Intelligence (AI): All You Need to Know!
Blogs / Trendy Tech Talks / What is Multimodal Artificial Intelligence (AI): All You Need to Know!
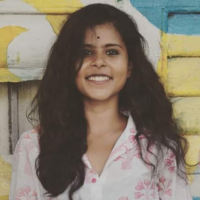
Ananya Dasgupta
04 Jun 2024
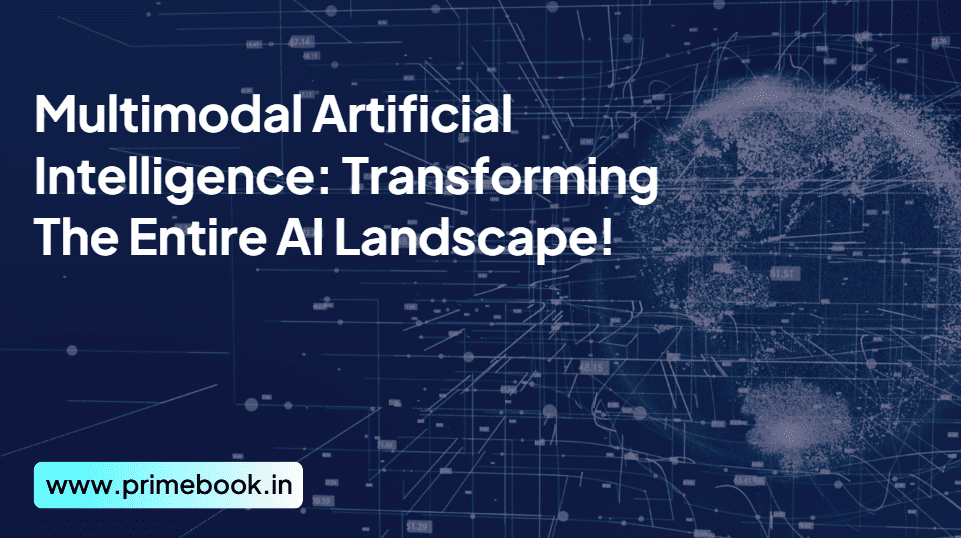
What is Multimodal Artificial Intelligence (AI): All You Need to Know!
Table of Contents
The boons of artificial intelligence are in word-of-mouth everywhere across the world. AI technology has become so advanced that it can automate a whole range of processes; not just creating some marketing campaigns. However, while a majority of the AI models work on a single data type, AI researchers and developers have brought a more robust solution, multimodal artificial intelligence (AI). This blog shall shed light on what multimodal AI is and how it’s changing the world of AI.
What is Multimodal AI?
As the name suggests, multimodal AI is a new and advanced type of artificial intelligence that combines multiple data types or modes to curate more accurate determinations, make more precise predictions, and draw more insightful conclusions about real-world problems.
This AI technology relies on data fusion techniques, whether early, mid, or late, and leverages the power of deep learning, natural language processing, computer vision (also called image analysis), audio processing, etc.
How Does Multimodal AI Work?
Multimodal AI follows a similar approach established on any AI and machine learning model, i.e., the algorithms that define the process in which data is learned and interpreted and subsequently responses are generated based on those data.
After the response gets generated, the output alongside the users’ approval or feedback is looped back into the model to stimulate continuous refinements and improvements within the model.
A series of 3 main components altogether build a multimodal AI, and they are – The Input Module, the Fushion Module, and the Output Module. In doing so, this technology can more accurately simulate human perception!
Generative AI & Multimodal AI: Differences!
The use of machine learning models to generate new content, such as images, music, texts, audio, and video typically from a single type of prompt comes under the umbrella term, Generative AI.
Whereas, multimodal AI adds on those generative capabilities by processing information from multiple modalities, like images, texts, videos, etc. It’s like giving AI the ability to process and understand diverse sensory modes.
It means that by availing of multimodal AI, users are no longer limited to one input or output type. They can prompt an AI model with virtually any input to curate virtually any output type. Google Gemini is one of the best examples in this regard!
Use Cases of Multimodal AI
Multimodal AI has a wide range of use cases. The most significant ones are as follows!
1. Text summarization
2. Visual information seeking
3. Object recognition
4. Digital content understanding
5. Structured content generation
6. Captioning and description
7. Long-form content
8. Reasoning and classification
9. Coding generation
10. Audio and video generation
11. Q/A and extrapolation
12. Entity extraction
13. Sentiment analysis
14. Understanding screen and interface
15. Understanding technical diagrams
16. Get recommendations or suggestions based on multiple images and more!
Benefits of Multimodal AI: What’s the Future?
Expanding the AI functionalities within the next-gen applications, multimodal AI represents a step forward in futurist technological progress. With more advanced reasoning, problem-solving, and generation abilities, this new AI technology enhances human-computer interaction. For example, you can use text or voice prompts to extract text from a particular image or covert image text in JSON and generate requisite answers related to the uploaded image.
Multimodal AI improves decision-making, drives innovation, and offers a more thorough and subtle understanding of various data. Enhanced contextual understanding is another salient benefit of this technology, which paves the way for more precise responses. It can even increase system performance in the face of unseen data, creating scopes for developing more robust AI models.
Challenges of Multimodal AI
1. As one of the latest technology developments, multimodal AI needs more improvement and improvisation to unleash its complete automation benefits for the world. This is because –
2. While datasets in multimodal AI involve aggregation from diverse sources, biases become an issue sometimes.
3. The neural networks developed through training within this technology can be difficult to interpret and infer for determining how exactly the AI calculates data and makes decisions. Here, the unpredictable nature of the model stands as a barrier to fixing bugs or eliminating data biases.
4. Besides, developers of multimodal AI need to find ways to overcome the nuances of training AI to distinguish between different meanings from an identical input. Training the AI model to align meaningful data from various data types stands vital herein!
To sum up, multimodal AI systems hold the potential to empower various industries to enhance their workflow and revenue. It can transform the way you interact with machines and experience the world around you. However, for that, it requires proper implementation and resolution of data complexities, privacy concerns, and data or decision-making biases. The future awaits to witness a powerful and purposeful side of multimodal AI due to its integration with IoT (Internet of Things).